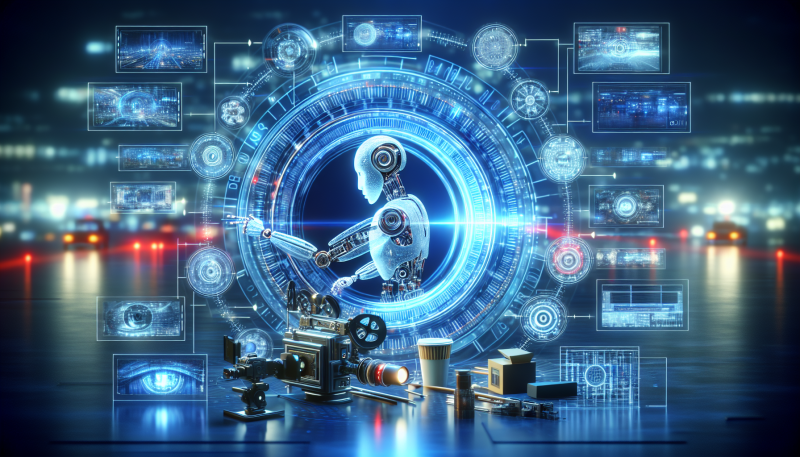
AI in Video Content Creation
In recent years, data science has emerged as a transformative force in various industries, and healthcare is no exception. By leveraging advanced analytics, machine learning, and artificial intelligence (AI), data science is revolutionizing the way healthcare providers diagnose, treat, and manage patient care. This comprehensive blog post delves into the myriad ways data science is enhancing healthcare, from improving patient outcomes to streamlining operations and fostering innovation.
The healthcare industry is undergoing a significant transformation, driven by the integration of data science and AI technologies. These advancements are not only improving patient care and diagnosis but also optimizing operational efficiencies and reducing costs. As healthcare providers increasingly adopt data-driven approaches, the potential for enhanced patient outcomes and more personalized care becomes ever more attainable.
In this blog post, we will explore the various applications of data science in healthcare, including predictive analytics, personalized medicine, medical imaging, and more. We will also discuss the challenges and ethical considerations associated with the use of data science in healthcare, as well as the future prospects of this rapidly evolving field.
Predictive Analytics in Healthcare
Predictive analytics is one of the most promising applications of data science in healthcare. By analyzing historical data and identifying patterns, predictive analytics can forecast future events and trends, enabling healthcare providers to make more informed decisions.
Early Disease Detection
One of the most significant benefits of predictive analytics is its ability to detect diseases at an early stage. By analyzing patient data, such as medical history, genetic information, and lifestyle factors, predictive models can identify individuals at high risk of developing certain conditions, such as diabetes, heart disease, or cancer. Early detection allows for timely intervention, which can significantly improve patient outcomes and reduce healthcare costs.
Hospital Readmission Reduction
Hospital readmissions are a major concern for healthcare providers, as they can lead to increased costs and poorer patient outcomes. Predictive analytics can help identify patients at risk of readmission by analyzing factors such as previous hospitalizations, comorbidities, and social determinants of health. By targeting these high-risk patients with tailored interventions, healthcare providers can reduce readmission rates and improve overall patient care.
Resource Optimization
Predictive analytics can also be used to optimize resource allocation within healthcare facilities. By forecasting patient demand and identifying trends in patient flow, healthcare providers can better manage staffing levels, bed availability, and other resources. This can lead to more efficient operations, reduced wait times, and improved patient satisfaction.
Personalized Medicine
Personalized medicine, also known as precision medicine, is an approach to healthcare that tailors treatment plans to individual patients based on their unique genetic makeup, lifestyle, and environmental factors. Data science plays a crucial role in enabling personalized medicine by analyzing vast amounts of data to identify the most effective treatments for each patient.
Genomic Data Analysis
Advancements in genomic sequencing have generated an enormous amount of data that can be used to understand the genetic basis of diseases. Data science techniques, such as machine learning and AI, can analyze this genomic data to identify genetic mutations and variations associated with specific conditions. This information can then be used to develop targeted therapies that are more effective and have fewer side effects than traditional treatments.
Pharmacogenomics
Pharmacogenomics is the study of how an individual’s genetic makeup affects their response to medications. By analyzing genetic data, healthcare providers can predict how patients will respond to different drugs and tailor their treatment plans accordingly. This can help avoid adverse drug reactions, improve treatment efficacy, and reduce trial-and-error prescribing.
Predictive Modeling for Treatment Outcomes
Data science can also be used to develop predictive models that forecast treatment outcomes based on patient-specific factors. These models can help healthcare providers identify the most effective treatment options for each patient, leading to better outcomes and more personalized care.
Medical Imaging and Diagnostics
Medical imaging is a critical component of modern healthcare, providing valuable insights into the diagnosis and treatment of various conditions. Data science is revolutionizing medical imaging by enhancing image analysis, improving diagnostic accuracy, and enabling the development of new imaging techniques.
Image Analysis and Interpretation
Traditional medical image analysis relies on the expertise of radiologists and other specialists to interpret images and identify abnormalities. However, this process can be time-consuming and subject to human error. Data science techniques, such as deep learning and computer vision, can automate image analysis and improve diagnostic accuracy. For example, AI algorithms can analyze medical images to detect tumors, fractures, and other abnormalities with high precision, often outperforming human experts.
Development of New Imaging Techniques
Data science is also driving the development of new imaging techniques that provide more detailed and accurate information about the human body. For example, machine learning algorithms can be used to enhance the resolution of medical images, allowing for better visualization of small structures and subtle abnormalities. Additionally, data science can help develop new imaging modalities, such as functional imaging, which provides insights into the physiological processes underlying various conditions.
Integration of Multimodal Data
Medical imaging is just one piece of the puzzle when it comes to diagnosing and treating patients. Data science can integrate multimodal data, such as medical images, electronic health records (EHRs), and genomic data, to provide a more comprehensive understanding of a patient’s condition. This holistic approach can lead to more accurate diagnoses, better treatment planning, and improved patient outcomes.
Electronic Health Records (EHRs) and Data Integration
Electronic health records (EHRs) are digital versions of patients’ medical histories, containing information such as diagnoses, treatments, lab results, and more. EHRs have the potential to improve patient care by providing healthcare providers with easy access to comprehensive and up-to-date patient information. However, the sheer volume and complexity of EHR data can be challenging to manage and analyze. Data science techniques can help unlock the full potential of EHRs by enabling efficient data integration, analysis, and visualization.
Data Integration and Interoperability
One of the key challenges in healthcare is the integration of data from various sources, such as EHRs, medical imaging systems, and wearable devices. Data science can facilitate data integration and interoperability by developing standardized data formats and protocols, enabling seamless data exchange between different systems. This can lead to more comprehensive and accurate patient records, improving care coordination and decision-making.
Natural Language Processing (NLP) for EHR Analysis
Much of the information in EHRs is stored in unstructured text, such as clinical notes and patient narratives. Natural language processing (NLP) is a branch of AI that focuses on analyzing and understanding human language. NLP techniques can be used to extract valuable insights from unstructured EHR data, such as identifying trends in patient symptoms, predicting disease progression, and detecting potential adverse events.
Predictive Analytics for Population Health Management
Data science can also be used to analyze EHR data at the population level, enabling healthcare providers to identify trends and patterns in patient populations. Predictive analytics can help identify high-risk patients, monitor disease outbreaks, and develop targeted interventions to improve population health. This can lead to more proactive and preventive care, ultimately reducing healthcare costs and improving patient outcomes.
Wearable Devices and Remote Monitoring
Wearable devices, such as fitness trackers and smartwatches, have become increasingly popular in recent years. These devices can collect a wealth of health-related data, such as heart rate, activity levels, and sleep patterns. Data science can analyze this data to provide valuable insights into patients’ health and enable remote monitoring and personalized care.
Continuous Health Monitoring
Wearable devices enable continuous health monitoring, providing real-time data on patients’ vital signs and activities. Data science techniques can analyze this data to detect anomalies and identify early warning signs of potential health issues. For example, machine learning algorithms can analyze heart rate data to detect irregularities that may indicate atrial fibrillation or other cardiac conditions. Early detection allows for timely intervention, potentially preventing serious health complications.
Personalized Health Recommendations
Data science can also be used to develop personalized health recommendations based on data collected from wearable devices. By analyzing patterns in activity levels, sleep quality, and other health metrics, data science algorithms can provide tailored advice on lifestyle changes, exercise routines, and other interventions to improve overall health and well-being.
Remote Patient Monitoring
Remote patient monitoring (RPM) is an emerging trend in healthcare that leverages wearable devices and data science to monitor patients’ health outside of traditional clinical settings. RPM can be particularly beneficial for managing chronic conditions, such as diabetes, hypertension, and heart disease. By continuously monitoring patients’ health data, healthcare providers can detect changes in their condition and intervene promptly, potentially preventing hospitalizations and improving patient outcomes.
Challenges and Ethical Considerations
While data science holds great promise for improving healthcare, it also presents several challenges and ethical considerations that must be addressed.
Data Privacy and Security
The collection and analysis of health data raise significant privacy and security concerns. Ensuring the confidentiality and integrity of patient data is paramount, as breaches can have serious consequences for individuals and healthcare organizations. Data science practitioners must adhere to strict data privacy regulations, such as the Health Insurance Portability and Accountability Act (HIPAA), and implement robust security measures to protect patient data.
Bias and Fairness
Data science algorithms can inadvertently perpetuate biases present in the data used to train them. For example, if a predictive model is trained on data that disproportionately represents certain demographic groups, it may produce biased predictions that disadvantage underrepresented populations. Ensuring fairness and equity in data science applications requires careful consideration of data sources, algorithm design, and evaluation metrics.
Transparency and Interpretability
Many data science algorithms, particularly those based on deep learning, are often described as “black boxes” due to their complexity and lack of interpretability. In healthcare, it is crucial for healthcare providers to understand how and why a particular prediction or recommendation was made. Developing transparent and interpretable models is essential for building trust and ensuring that data science applications are used responsibly in clinical decision-making.
Regulatory and Ethical Compliance
The use of data science in healthcare is subject to various regulatory and ethical guidelines. Ensuring compliance with these regulations is essential to protect patient rights and maintain public trust. Additionally, ethical considerations, such as informed consent and the responsible use of AI, must be carefully addressed to ensure that data science applications are used in a manner that respects patients’ autonomy and well-being.
Future Prospects of Data Science in Healthcare
The future of data science in healthcare is bright, with numerous exciting developments on the horizon. As technology continues to advance, the potential for data science to transform healthcare will only grow.
Integration of AI and Machine Learning
AI and machine learning are poised to play an increasingly prominent role in healthcare. From predictive analytics and personalized medicine to medical imaging and remote monitoring, AI-powered solutions have the potential to revolutionize patient care and diagnosis. As these technologies continue to evolve, we can expect to see even more sophisticated and effective applications of data science in healthcare.
Expansion of Wearable Devices and IoT
The proliferation of wearable devices and the Internet of Things (IoT) will generate vast amounts of health data that can be harnessed for improved patient care. Data science will play a crucial role in analyzing this data and providing actionable insights to healthcare providers and patients. The integration of wearable devices with EHRs and other healthcare systems will enable more comprehensive and personalized care.
Advancements in Genomics and Precision Medicine
As genomic sequencing becomes more affordable and accessible, the field of precision medicine will continue to expand. Data science will be instrumental in analyzing genomic data and identifying targeted therapies for various conditions. The integration of genomic data with other health data sources will enable more precise and effective treatments, ultimately improving patient outcomes.
Telemedicine and Remote Care
The COVID-19 pandemic has accelerated the adoption of telemedicine and remote care, highlighting the importance of data science in these areas. Data science can enhance telemedicine by providing real-time analytics, predictive modeling, and personalized recommendations. As telemedicine continues to grow, data science will play a key role in ensuring that remote care is effective, efficient, and patient-centered.
Conclusion
Data science is revolutionizing healthcare by improving patient care and diagnosis through predictive analytics, personalized medicine, medical imaging, and more. While there are challenges and ethical considerations to address, the potential benefits of data science in healthcare are immense. As technology continues to advance, data science will play an increasingly vital role in shaping the future of healthcare, leading to better patient outcomes and more efficient healthcare systems.
For healthcare providers and organizations looking to harness the power of data science, it is essential to stay informed about the latest developments and best practices. By embracing data-driven approaches and leveraging advanced analytics, healthcare providers can enhance patient care, optimize operations, and drive innovation in the industry.
To learn more about how AI and data science are transforming various industries, including healthcare, explore our blogs on the subject. Discover how AI is revolutionizing business blogging in our detailed post on How AI is Revolutionizing Business Blogging, and delve into the benefits of AI in digital content strategy with our insightful article on The Benefits of AI in Digital Content Strategy. Additionally, explore the future of SEO with AI in our comprehensive post on AI: The Future of SEO, and understand why AI is essential for business blogging in our detailed analysis on Why AI is Essential for Business Blogging.
Embrace the future of healthcare with data science and AI, and unlock the potential for improved patient care and diagnosis. Stay ahead of the curve and drive digital excellence in your healthcare organization by leveraging the power of data science and AI-powered tools.